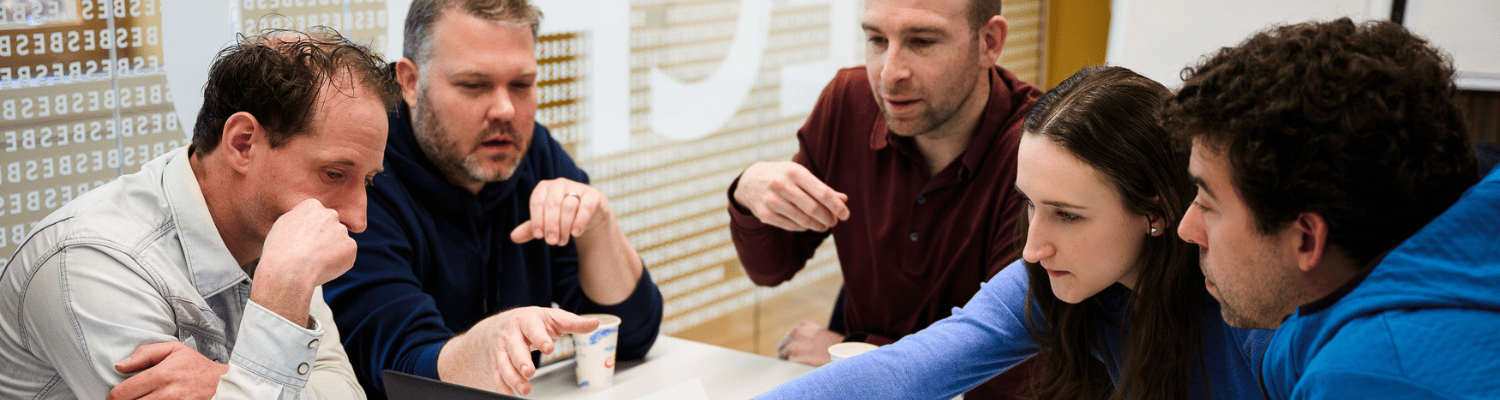
09 Mar The promise and challenges of data sciences
“I really enjoyed the Data Science Life Cycle session. It gave me the opportunity to familiarise with some of the challenges of data science projects. Also, I was stimulated to look at data dimensions in a different way. Often, we start looking at data sets with strong assumptions that are not necessarily true. We were presented with powerful analytical tools to challenge these assumptions”, says Davide Pellegatta (42), living in Luxemburg and working for Couchbase NoSql database.
The session Data Science Life Cycle, presented by Dr. Johan van Soest, is part of the Digital Management elective week, which is part of the Digital Business Course. 29 Students from all directions, both from the Online MBA track and the On-Campus MBA track, were immersed in the world of data science. Johan van Soest took off with the ‘buzz word bingo’, explaining the various concepts and abbreviations in the data science business. He also clarified the various types of data, how to capture and use them and some aspects of ‘big data’.
About Johan van Soest
Dr. Johan van Soest is assistant professor Translational Data Science at the Brightlands Institute for Smart Society (BISS). His background is in Medical Informatics, he holds a PhD in Clinical Data Science. His research concentrates on methods for information exchange and responsible application of artificial intelligence in practice. Specifically, his focus is on methods for making the data science process more aware of ethical, legal and societal aspects (ELSA) and making these considerations transparent to potential users of AI. Second, he is concerned with the translational gap between data science insight generation and implementation in (clinical) practice, addressing the question what is needed to use data science insights safely in (clinical) practice.
CRISP-DM method
Data Science and AI hold huge promises to improve business decision making by generating valuable insights. However, it’s not a magical tool, as Johan points out. One of the great challenges is to deal with the enormous amount of data and organising the data pipeline from raw data to relevant management information. The CRISP-DM method (Cross-Industry Standard Process for Data Mining) is a method often used. During the session the students deep-dived into this method. CRISP-DM is a process-based approach that helps businesses to define the problem, to collect, understand and analyse the data suited for their purpose and evaluating the results.
Six steps
Step 1 in the Data Science Life Cycle is business understanding or, what is the problem that needs to be solved. The second step, data understanding, is about considering the data, for what purpose were they originally collected, how do they link to the business problem, how relevant are they and what are the costs and benefits of retrieving them. Step 3 is data preparation; making sure the data are correct. The data is then ready to be modeled (step 4). In this step one should consider which tool is best fitted for answering the question. The fifth step is evaluation. Are the results valid and reliable, does the chosen model fit the original business goal, how good are the predictions? The final step is deployment; introducing and using models in real-life.
Conveying the complexity
Davide: “As a former developer data science wasn’t new to me, but yet I never dug in deep enough. Therefore, my next step is to dedicate some time to this topic. To me, this session had a big role in conveying the complexity behind engineering choices that sometimes elude the management. I really enjoyed it.”
This article displays the student insights and experiences of the Digital Management module of the Digital Business elective. The MaastrichtMBA programme has an executive modular part-time format and offers two tracks: On-Campus MBA and Online MBA. The programme has a Triple Crown accreditation and is aimed for professionals with at least 5 years of working experience.